ASPiRE
Xen.AI ASPiRE
AI as a Key to Sustaining Quality Amidst the Radiologist Shortage
This paper addresses the challenges radiologists face in maintaining report quality amidst these pressures and explores how artificial intelligence (AI), in a vendor-neutral framework, is emerging as a solution to enhance report accuracy, radiologist education, and overall quality of care.
The Crisis in Radiology: Shortages and Rising Workloads
The current radiologist shortage is not a temporary issue; it is a systemic challenge that shows no signs of abating. According to a report by the American College of Radiology, more than half of radiologists report that their workloads have increased substantially over the past five years, a trend that is expected to continue. This shortage is further compounded by the aging population, which demands more imaging services, and the rapid advancement of imaging technologies that produce increasingly complex cases.
As radiologists grapple with these overwhelming workloads, maintaining the quality of their reports has become a Herculean task. For many, the pressure to meet turnaround times and keep up with case volumes leads to compromises in thoroughness and diagnostic precision. The long hours, repetitive tasks, and lack of time for reflection and learning result in radiologists being trapped in a cycle of efficiency over excellence.
The Consequences of Diminished Report Quality
The implications of these challenges are profound. Diagnostic errors in radiology not only delay patient care but can lead to incorrect treatments, increased healthcare costs, and, most importantly, harm to patients. Radiology departments are often judged by their ability to provide quick, reliable interpretations. However, when the emphasis shifts entirely to speed, there is a risk that the accuracy and depth of radiological reports may suffer. Additionally, radiologists under pressure are missing key opportunities for growth and development. Continuing education is essential for staying updated with the latest advances in imaging technology, new diagnostic criteria, and evolving best practices. Unfortunately, the relentless pace of the workload leaves little time for these educational pursuits.
AI: A Bridge Between Efficiency, Education, and Quality
This is where AI can play a pivotal role. While not a replacement for radiologists, AI tools are emerging as valuable allies in enhancing the accuracy and quality of radiology reports. AI-driven systems can automate time-consuming tasks, assist in identifying subtle abnormalities, and provide instant feedback on potential errors, allowing radiologists to focus on more complex cases and educational growth.
Value-Based Care and AI’s Role in Radiology
In the context of value-based care, where patient outcomes are directly tied to the quality of care provided, the stakes for radiology have never been higher. Poor-quality radiology reports can lead to incorrect diagnoses, inappropriate treatment plans, and increased costs from unnecessary follow-up procedures. As healthcare systems increasingly adopt value-based models, radiologists will need to ensure that their work not only meets the demands of efficiency but also adheres to the highest standards of accuracy and quality.
AI is becoming an essential tool in aligning radiology practices with the goals of value-based care. By improving diagnostic accuracy and ensuring continuous learning, AI helps radiologists provide more precise, reliable diagnoses. This, in turn, improves patient outcomes, reduces diagnostic errors, and lowers the overall cost of care—goals that are central to the value-based care framework.
The Subtle Role of AI in Peer Review
While peer review has always been an integral part of ensuring quality in radiology, it is not without its limitations. Traditional peer review is often subjective, time-consuming, and constrained by the availability of expert reviewers. AI can address these challenges by introducing objectivity and scalability to the peer review process. AI-driven peer review systems can automatically flag discrepancies, compare reports against established standards, and provide data-driven insights to both the reviewing radiologist and the original report author.
It’s important to note that the introduction of AI in peer review should not be seen as a replacement for human expertise, but as a tool that enhances the process. Radiologists remain in control of the final interpretation and report but are supported by AI’s ability to provide more consistent, reliable feedback. This integration of AI into peer review can help radiologists catch errors early, learn from their mistakes, and ultimately improve the quality of their work.
Conclusion:
AI as a Key to Sustaining Quality Amidst the Radiologist Shortage
The current state of radiology—marked by rising workloads, a shortage of professionals, and the pressure to maintain efficiency—presents significant challenges to the quality of patient care. However, AI offers a promising solution. By automating routine tasks, enhancing diagnostic accuracy, providing targeted education, and supporting the peer review process, AI can help radiologists maintain the highest standards of quality while managing increasing demands.
In this evolving healthcare landscape, radiologists who embrace AI will not only be able to keep pace with the demands of their profession but also enhance their skills, improve patient outcomes, and contribute to the future of value-based care. As AI continues to evolve, its role in radiology will become even more critical, ensuring that the quality of radiology reports does not diminish, even in the face of mounting pressures.
Know the expert
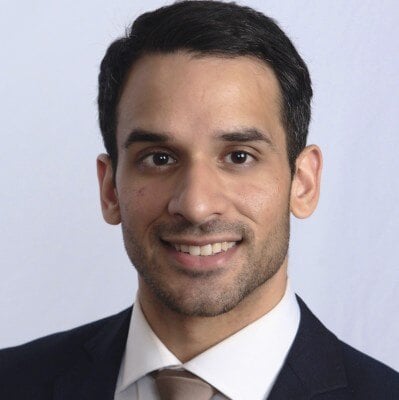
Talk to the expert
Extraordinary competence produces astonishing results.