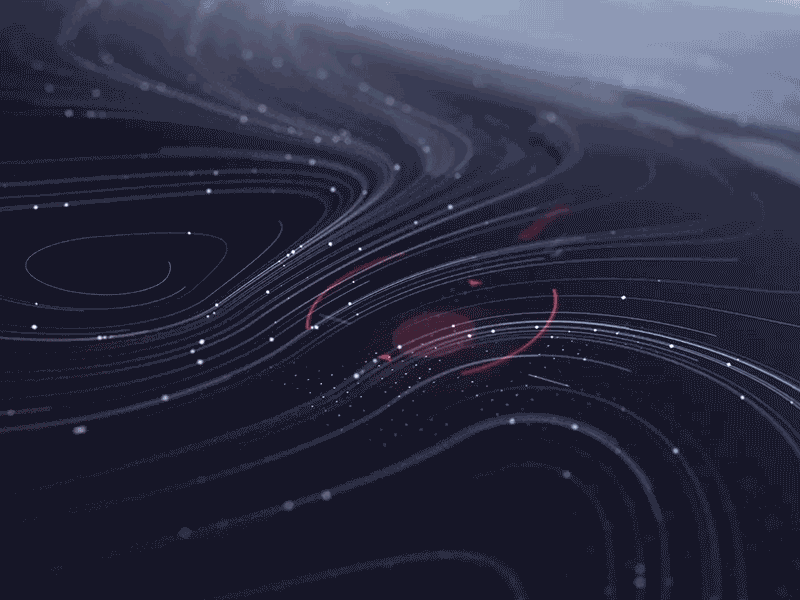
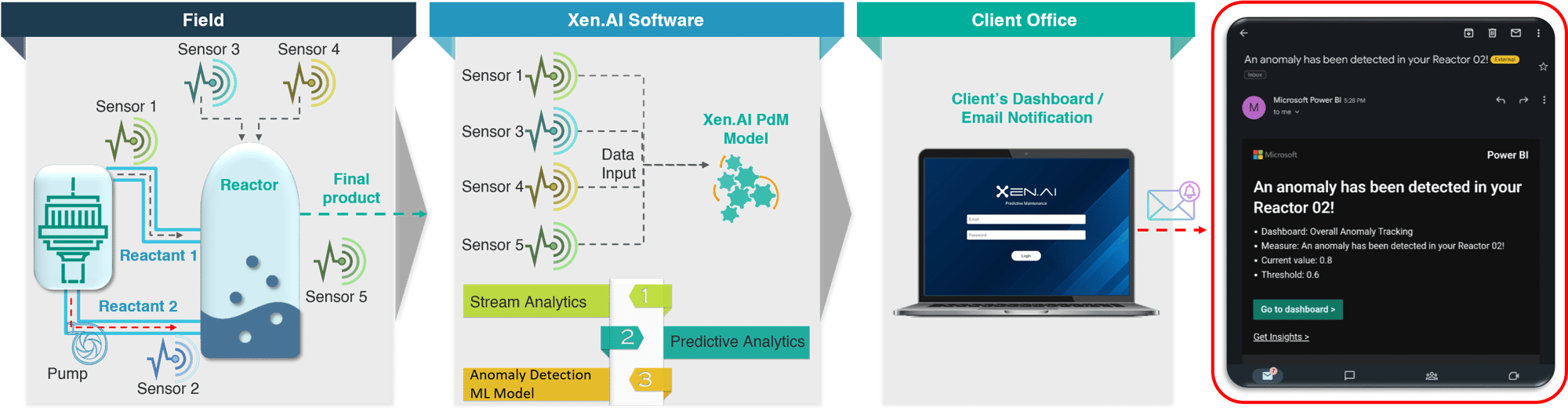
Features
Constantly monitoring the health status of equipment and processes can be challenging, as each process and its associated equipment generate lots of live sensor data. This monitoring gets even more complex when these sensor data have an implicit relationship with each other and need to be monitored and analyzed together. Defining monitoring rules for this complicated relationship between the sensors manually can be costly and inefficient.Traditionally, manufacturing facilities have solved this problem through first principles models and the operational knowledge of experienced operators. The first principle approach tends to only focus on individual key sensors and create a setpoint or threshold for the few key sensors to alarm when the setpoint is exceeded. The problem with this approach is that other equipment and process components are left out of the monitoring process since including them will result in too many alarms and sensors to be monitored by a few operators. Furthermore, modeling all the complicated relationships between all the sensors via a first-principles methodology to determine the proper alarm threshold is just impractical. The aging workforce, retirements, employee turnover, and human physical limitations also make relying solely on experienced operators, not a sustainable long-term solution. These traditional approaches have often led to "sudden" major equipment failures, out-of-specification products that sneaked up on operations, major maintenance downtimes and in some cases permanent manufacturing unit shutdowns.
Xen.AI Anomaly Detection and Predictive Maintenance (PdM) uses machine learning and artificial intelligence to detect possible system failures before they occur. Our PdM software analyzes real-time data from sensors and alerts operators to take proactive actions as soon as early signs of failure or process anomalies are detected. This data-driven approach helps minimize downtime, reduce maintenance costs, prevent out-of-specification final products and improve overall operational efficiency by notifying operations to immediately investigate and resolve anomaly issues before they escalate.
In short, this is an extra set of sharper eyes to monitor the condition of your equipment and processes. You can call it your "Co-Operator". We harness the capabilities of cloud computing (Azure and AWS) on the backend, ensuring the utmost level of data security.
Uses
Xen.AI PdM is an effective way of avoiding industrial machine failures and expensive downtime by proactively monitoring the equipment
Xen.AI PdM uses historical data from sensors and transmitters to train a model tailored to the specific operational patterns of your process equipment in a healthy state.PdM software analyzes incoming operational data in real time and alerts you as soon as it identifies signs of anomaly.
Not only does the software monitor the individual sensors but it also tracks the intricate relationships and patterns among all the sensors.
Quickly detects hard-to-find anomalies.
The software displays sensors that are contributing to the anomaly, thereby facilitating troubleshooting.
Benefits
Xen.AI PdM solution can be helpful in a wide variety of industry fields, for example, automotive, railway, manufacturing, oil and gas, infrastructure management, etc. Possible positive outcomes include:Ability to predict and monitor potential maintenance or failures and plan all related actions in advance.
Make very specific part replacements rather than a costly bulk screening of all potential causes.
Timely detection of failures can help reduce the amount of unplanned downtime repairs and relevant costs.
Increase customer satisfaction and retention.
Increase the company revenue.
Talk to an expert
Extraordinary competence produces astonishing results.